Introduction to paralel and distributed computing
Contents
Introduction to paralel and distributed computing#
1. A simple computation problem#
Final GPA calculation
Components of a computation problem
Computational task
Execution framework.
Computing resources.
Computational tasks should be able to …
Be borken apart into discrete pieces of work that can be solved simultaneously.
Be solved in less time with multiple computing resources than with a single computing resource.
Execution framework should be able to …
Execute multiple program instructions concurrently at any moment in time
Computing resources might be …
A single computer with multiple processors.
An arbitrary number of computers connected by a network.
A special computational component inside a single computer, separate from the main processors (GPU), or
Any combintations of the above.
Parallelizing final GPA calculations
2. Parallel and distributed computing systems#
Definition
A collection of individual computing devices that can communicate with each other. (Attiya and Welch, 2004)
Emphasis …
A collection of individual computing devices that can communicate with each other. (Attiya and Welch, 2004)
How do parallel and distributed computing resources evolve?
Single site, single computer, single core
Single site, single computer, multiple cores
Single site, multiple computers, multiple cores
Cluster computing
Multiple sites, multiple computers, multiple cores, federated domains
Grid computing
Multiple site, multiple computers, multiple cores, virtula unified domain
Cloud computing
3. Can we just throw more computers at the problem?#
Definitions
Parallel speedup: how much faster the program becomes once some computing resources are added.
Parallel efficiency: Ratio of performance improvement per individual unit of computing resource.
Parallel speedup
Given
p
processors,Speedup,
S(p)
, is the ratio of the time it takes to run the program using a single processor over the time it takes to run the program usingp
processors.The time it takes to run the program using a single processor, \(t_{s}\): sequential run time
The time it takes to the the program using multiple processor, \(t_{p}\): parallel run time
\(S(p) = \frac{sequential\ run\ time}{parallel\ run\ time} = \frac{t_s}{t_p}\)
Example 01
A program takes 30 seconds to run on a single-core machine and 20 seconds to run on a dual-core machine. What is the speedup of this program?
Solution
\(t_s=30\)
\(t_p=20\)
\(S=\frac{t_s}{t_p}=\frac{30}{20}=1.5\)
Theoretical max
Let
f
be the fraction of the program that is not parallelizable.Assume no overhead.
Running the program using one processor will take time \(t_s\).
The parallel run time, \(t_p\), can be calculated as the time it take to run the fraction that is non-parallelizable (\(f\times t_s\)) plus the remainning parallelizable fraction (\(1-f\)).
If \(p=1\), this simplifies to \(t_p=f\times t_s + (1-f)\times t_s\).
Assume no overhead, this means that we reduce the speed by half as we double the number of processor.
And so on …
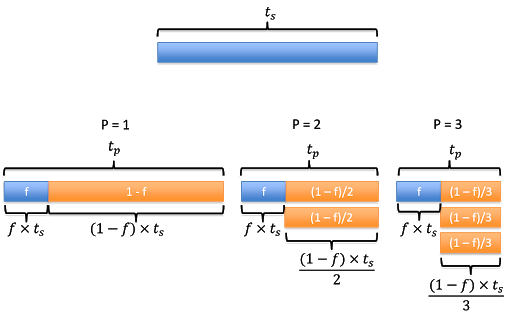
Amdahl’s Law
This brings us to Amdahl’s Law, which quantifies speedup in term of number of processors and fraction of non-parallelizable code:
\(S(p)=\frac{t_s}{t_p}=\frac{t_s}{f\times t_s + (1-f)\times t_s}=\frac{1}{f + \frac{1-f}{p}}=\frac{p}{f \times (p-1) + 1}\)
Parallel efficiency
The efficiency
E
is then defined as the ratio of speedupS(p)
over the number of processorsp
.
\(E=\frac{\frac{p}{f \times (p-1) + 1}}{p}=\frac{1}{f \times (p-1) + 1}\)
E is often measured as percentage.
For example,
E = 0.8
means the parallel efficiency is 80%.
Example 02
Suppose that 4% of my application is serial. What is my predicted speedup according to Amdahl’s Law on 5 processors?
Solution
\(f=0.04\)
\(p=5\)
\(S=\frac{p}{(p-1)f + 1}=\frac{5}{4 \times 0.04 +1}=4.3103\)
Example 03
Suppose that I get a speedup of 8 when I run my application on 10 processors. According to Amdahl’s Law:
What portion of my code is serial?
\(S=8\)
\(p=10\)
\(S=\frac{p}{(p-1)f + 1}\)
\(8=\frac{10}{9f+1}\)
\(9f + 1 = \frac{10}{8}\)
\(f=\frac{1}{36}\)
What is the speedup on 20 processors?
\(f=\frac{1}{36}\)
\(p=20\)
\(S_{20}=\frac{p}{(p-1)f + 1}=\frac{20}{\frac{19}{36}+1} \approx 13.0909\)
What is the efficiency on 5 processors? 20 processors?
\(E=\frac{1}{(p-1)f + 1}\)
\(E_5=\frac{1}{\frac{4}{36}+1} = 90\% \)
\(E_{20}=\frac{1}{\frac{19}{36} + 1} \approx 65.45\% \)
What is the best speedup that I could achieve?
\(f=\frac{1}{36}\)
\(S_{\infty}=\lim_{p \to +\infty} \frac{p}{(p-1)f + 1} = \lim_{p \to +\infty} \frac{1}{\frac{p}{p-1}f + \frac{1}{p}}=\frac{1}{f}\)
\(S_{\infty}= 36\)
In other word, the highest number of processors one should add to this problem is 36.
Limiting factors of parallel speedup
Non-parallelizable code.
Communication overhead.
If there is no limiting factor …
0% non-paralellizable code.
No communication overhead.
\(S_{\infty}=\lim_{f \to 0} \frac{p}{(p-1)f + 1} = p\)
\(S \leq p\)
Superlinear speedup
The unicorn of parallel and distributed computing.
Poor sequential reference implementation.
Memory caching.
I/O blocking.
4. Parallel and distributed computing system architectures#
Types of distributed computing systems
Streaming SIMD extensions for x86 architectures.
Shared memory.
Distributed shared memory.
Heterogeneous computing (accelerators).
Message passing.
Shared memory
Heterogeneous computing
GPU
FPGA
Co-processors
GPU - graphics processing unit
Processor unit on graphic cards designed to support graphic rendering (numerical manipulation).
Significant advantage for certain classes of scientific problems.
Programming models:
CUDA: Library developed by NVIDIA for their GPUs.
OpenACC: Standard developed by NVIDIA, Cray, and Portal Compiler (PGI).
OpenAMP: Extension to Visual C++ to direct computation to GPU.
OpenCL: Public standard by the group the developed OpenGL.
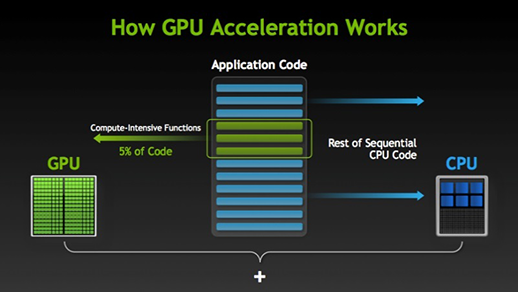
FPGA - field programmable array
Dynamically reconfigurable circuit board.
Expensive, difficult to program.
Power efficient, low heat.
Co-processors
Enables offloading of computationally intensive tasks from main CPU.
Similar to GPU, but can support a wider range of computational tasks.
Intel
Xeon Phi processor line.
PCIe-based add-on cards, but could also be used as a stand alone CPU.
Unlike GPU, Intel Xeon supports all programs targeted to standard x86 CPU (very minor modification if any)
Message passing distributed computing
Processes handle their own memory.
Data is passed between processes via messages.
Scales well.
Cluster can be built from commodity parts.
Cluster can easily be expanded.
Cluster can be heterogeneous.
Programming models:
MPI: standardized message passing library.
MPI + OpenMP: hybrid model.
MapReduce programming model for big data processing.
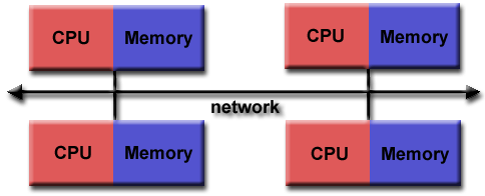
5. Benchmarking#
Benchmarking suites
LINPACK (Linear Algebra Package): Dense Matrix Solver
HPCC: High-Performance Computing Challenge.
HPL (LINPACK to solve linear system of equations)
DGEMM (Double precision general matrix multiply)
STREAM (Memory bandwidth)
PTRANS (Parallel matrix transpose to measure processors communication)
RandomAccess (random memory updates)
FFT (double precision complex discrete fourier transform)
Communication bandwidth and latency
SHOC: Scalable heterogeneous computing
Non-traditional system (GPU)
TestDFSIO
I/O performance of MapReduce/Hadoop Distributed File System.
Ranking systems
TOP500: Rank the supercomputers based on their LINPACK score.
GREEN500: Rank the supercomputers with emphasis on energy usage (LINPACK/power consumption).
GRAPH500: Rank systems based on benchmarks designed for data-intensive computing.